1. Introduction
Language barriers and disparities in multilingualism has been widespread issues for many decades, and it has been impacting individuals and communities globally. In today’s interconnected world, importance of cross-cultural communication is growing ever more essential due to globalization and the ability to speak and understand multiple languages hold significant importance. On the other hand, multilingual ailibies are not uniform across populations, causing unequal access to education, employment, healthcare, and other social opportunities (Kaplan & Haenlein 2019, Gleason 2022, Hirsh-Pasek & Blinkoff 2023, Shinde 2023). In addition, the advancement of AI1 technologies has resulted in transformative changes across diverse domains, promising increased efficiency, accuracy, and accessibility. However, amidst this technological progress, a critical issue emerges: the exacerbation of biases against non-native English speakers. AI systems predominantly operate within the framework of English-centric datasets and models, they often struggle to effectively interpret and respond to linguistic variations, accents, and dialects prevalent among non-native English speakers. Consequently, non-native English speakers encounter systemic biases, leading to disparities in access to AI-powered services, including voice recognition systems, language translation tools, and automated decision-making processes (Bhirud et al. 2019, Bozkurt & Sharma 2020, Frangoudes et al. 2021, Biswas 2023, Cascella et al. 2023, Fütterer et al. 2023, Gilson et al. 2023).
Such biases not only impede the full integration of non-native English speakers into the digital landscape but also sustain linguistic inequalities in the realm of AI-driven technologies. Therefore, it is imperative to critically examine the mechanisms through which AI advancements engender biases against non-native English speakers and explore strategies to mitigate these disparities, fostering a more inclusive and equitable AI ecosystem for all users. Thus, the current paper tries to provide comprehensive reviews of AI chatbots and thier effects in existing industries and some biases caused by advancement of the system. In Section 2, we will firstly review the studies on the development of large language models and its effects to education and healthcare systems. Section 3 will discuss studies on the potential disadvantages and baises caused by the AI chatbot system to the non-native English speakers. Section 4 concluds the study by suggesting possible solutions.
2. AI and New Realm
The world has changed significantly for the past few decades and the change is ongoing. One notable development that is causing a lot of interest in academia is the emergence of LLM such as ChatGPT, a NLP model developed by OpenAI (Shinde 2023). The model functions on extensive datasets, and it can respond to students’ questions, feedback, and prompts (Biswas 2023, Gilson et al. 2023). In education, there has been increasing number of studies on the benefits and challenges of using chatbots (Aydın & Karaarslan 2022, Stokel-Walker 2022, Adeshola & Adepoju 2023, Bonsu & Baffour-Koduah 2023, Fütterer et al. 2023, Hirsh-Pasek & Blinkoff 2023). Some educators are optimistic about its potential to aid learning (Bonsu & Baffour-Koduah 2023). According to the research, one of the key applications of ChatGPT in the classroom is personalized learning opportunities. This entails developing educational resources and content specifically tailored to each student’s individual interests, skills, and learning objectives (Bonsu & Baffour-Koduah 2023).
Others express concerns about its potential to generate learning opportunities or perpetuate misinformation (Fütterer et al. 2023). Fütterer and his colleagues analyzed Twitter data (16,830,997 tweets from 5,543,457 users) to understand reactions about ChatGPT concerning education. Based on topic modeling and sentiment analysis, they provided an overview of comprehensive perceptions and reactions to the chatbot. As one might expect, the chatpot triggered a massive response on Twitter, and ‘education’ was the most tweeted content topic, surpassing more general topics such as how to access ChatGPT. The topics include from specific terms such as ‘cheating’ to broad ones such as ‘opportunities’ and they were discussed with mixed sentiments.
According to the authors, it is surprising and meaningful because the platform could considerably modify professional practice in many fields, which centers on creative text production, such as journalism, book authoring, marketing, and business reports. It implies that educational stakeholders like school and higher education administrators, teachers, and policymakers should formulate guidelines to impelement the platform within their respective enviroenments.
The emergence of ChatGPT and similar AI chatbots has shed light on the vulnerability of the educational system to external threats (Bozkurt & Sharma 2020). These AI tools could potentially be utilized for cheating on exams or completing assignments without genuine effort, as they can deliver responses instantly upon demand. This not only compromises the integrity of the educational system but also puts students at a disadvantage if they lack access to such resources, especially when instructors are unaware of their usage and inadvertently rate those who use them higher.
Moreover, accrding to Hirsh-Pasek & Blinkoff (2023), the landscape of higher education has become increasingly competitive as shown in other industries. A multitude of universities and colleges now offer similar programs and cost structures, necessitating institutions to distinguish themselves and craft compelling brand identities to attract students. Hirsh-Pasek & Blinkoff (2023) argues that it is imperative that universities and colleges also ensure prospective students understand the unique benefits of enrolling with them. While some universities advocate for the integration of AI in education, others oppose it, resulting in a lack of consensus on its usage in higher education. Therefore, it’s essential for educators to model exemplary behavior (Hirsh-Pasek & Blinkoff 2023). As we have reviewed, the impact of LLM like ChatGPT in education is enormous.
Another field that has been heavily affected by the extensive large language processing models includes healthcare system. It was shown that 60% of doctor visits are for minor diseases, and 80% of them can be treated at home by using simple remedies (Bhirud et al. 2019, Frangoudes et al. 2021, Cascella et al. 2023). These diseases usually include cold, cough, headache, abdominal pains and so on. They are often known to be attributed to factors such as weather changes, poor nutrition, and fatigue, which can be managed without medical intervention (Cascella et al. 2023).
Chatbots can assist potential patients by offering basic healthcare information before they seek to make an appointment with doctors. It can predict the users’ diseases based on symptoms and offers recommendations for precautions and remedies. If a severe illness is suspected, the device can advise users to seek medical assistance. Its primary goal is to communicate with the user in a manner like that of a doctor so that users can freely discuss any problems they may be experiencing. Acting as a virtual friend, the system aims to facilitate healthcare couseling to potential patients.
In addition, an increasing number of studies claim that LLMs can benefit mental healthcare systems (Aydın & Karaarslan 2022, Ayers et al. 2023, Kanjee et al. 2023, Sharma et al. 2023, Shryock 2023). That is because unlike search engines, which provide responses and links when they receive text inputs, chatbots like GPT-4 deliver reponses that are similar to human conversations. In fact, as WHO (World Health Organization 2022) estimates, approximately one in eight individuals worldwide are going through mental illness. This issue is compounded by stigmatization, human rights violations, and insufficient resources. In particulary, shortages of mental healthcare professionals prevent the patients from accessing to psychiatric treatment. Therefore, as the time of clinicians is highly limited resource in mental healthcare, advancements in artificial intelligence may enhance the efficiency of clinicians, and assist with some administrative duties.
Given these challenges, recent progress in generative AI and its potential to influence healthcare delivery have gained significant interest. Some studies suggest that chatbots which are powered by extensive language models have the potential to aid mental health peers and clinicians by consistently providing high levels of support during interactions with patients. For instance, one of the studies revealed that responses developed in collaboration with a chatbot named ‘HAILEY’ were more likely to be perceived as emphatic compared to responsos provided soley by humans (Sharma et al. 2023).
What is more, peer supporters who acknowledged difficulties in offering empathetic support were notably rated as more likely to deliver empathetic responses when supported by AI. In addition to aiding clinician documentation and patient interactions, an emerging strength of generative AI also lies in hypothesis generation. Preliminary studies demonstrate the potential of GPT-4 in generating accurate lists of potential diagnoses, particularly in complex clinical cases, indicating its ability to facilitate hypothesis formation (Ayers et al. 2023, Kanjee et al. 2023, Shryock 2023).
While acknowledging benefits of using generative AI chatbots, some risks of harm are also suggested (King 2022, Ferrara 2023, Gross 2023, Marks & Haupt 2023). In addition to the technological deficiencies commonly characterized by inconsistent responses and dissemination of false information, certain biases may also be observed, potentially leading to fatal outcomes. LLMs can write responses in a prompted conversational register such as tone or level. Nevertheless, due to a range of factors, biases are inherently ingrained, giving rise to the risk of ‘algorithmic discrimination’, and outcomes may sustain or worsen unfair treatment. Research indicates that these models can encode biases related to gender, race, and disability, jeopardizing their equitable implementation (King 2022, Ferrara 2023).
Ferrara (2023) explains that bias stems from mutliple sources. Training data often exhibit gaps in representation from clinical population, particularly in medical publications like PubMed. Moreover, stereotyping seems to emerge from diverse sources such as social media platforms including Twitter, Facebook, and among others. Furthermore, biases from people and society can get into the system through supervised learning.2 Workers, who often don’t get paid much, might continue unfair stereotypes when they label data and give feedback (Ferrara 2023).
As we have reviewed, Artificial Intelligence holds the potential to revolutionize industries such as education and healthcare, and results in advancements, creativity, and enhanced effectiveness. To summarize, in education, it can provide individualized learning opportunities and extend quality of education to distant areas. In terms of healthcare system, AI can aid in early detection of diseases and provide customized treatment strategies. While it tends to provide potentially advantageous landscape in such areas, there tends to exist some issues related to ‘bias’ issues in using the tool.
3. Biases to Non-Native English Speakers
In section 2, we have reviewed benefits of LLM in education and healthcare systems. However, the prevalent understanding is that AI-driven language technology which encompasses large language models, machine translation systems, multilingual dictionaries, and corpora, is presently limited to merely the world’s predominant languages, those that receive substantial financial and political backing. AI systems, particularly language models, heavily rely on a vast array of online data sources and corpora including forums, articles, and enclyclopedias, among many others. However, there seems to be notable imbalance in this digital landscape. That is to say that dominance of English language is overwhelming, whereas other languages are underrepresented.
Figure 1 shows the percentage of websites using a range of content languages. As the figure represents, among the websites whose content language we are aware of, English is utilized by 50.9%. This linguistic inequality suggests significant challenges in AI development and causes important issues about fairness and inclusiveness in the digital age. The widespread usage of English provides English native speakers with great advantages by providing easy access to abundant useful information and resources. Naturally, language models that are predominantly trained on English language data could exhibit more advanced and comprehensive understanding of data and nuanced capability in English language AI applications. In fact, if one tries to ask questions or exchanges conversations in English, it would give much more information than it was asked with other languages like Korean on the same topic. For example, when you ask about a simple question such as “Please provide me with features of rhinoceros beetles” in English, it would give you answers containing 8 categories of its characteristics in a well-organized format, which enhances readability. On the other hand, if it is asked the same question in Korean, it would give you the similar answer, but only containing 4 catetories of the features of the insect. Even though this is a simplistic example, it unequivocally demonstrates the disparity in the amount of information accessible when conversing in English compared to when conversing in other languages.
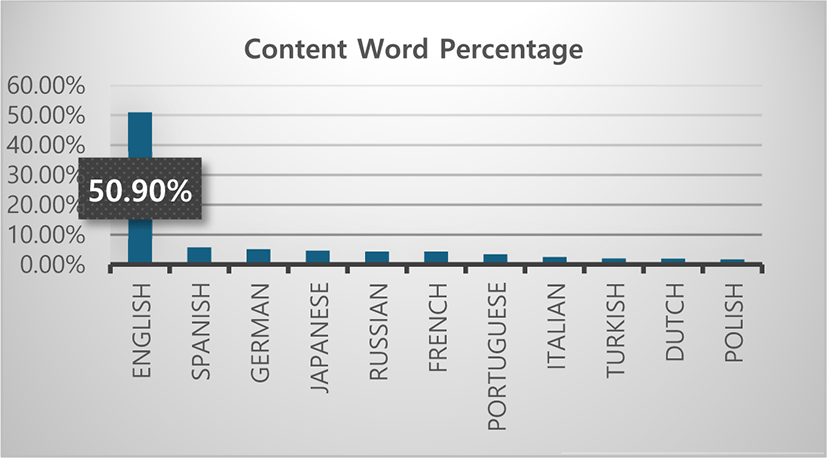
It is widely known that recent language models have improved significantly (Devlin et al. 2018, Brown et al. 2020, Clark et al. 2020). Recent advancements in language modeling have embraced the approach of training large-scale models on extensive, unannotated corpora using self-supervised learning techniques. These methods involve predicting masked words and the next sentence in a sequence. (Devlin et al. 2018, He et al. 2020), wrong word detection (Clark et al. 2020), and left-to-right language generation (Brown et al. 2020, Raffel et al. 2020).
The recent natural language processing models are trained by assessing the similarity vocabularies and sentences in text. Since the optimization objective focuses on maximizing the likelihood of the training data, the trained model enhances the coherence of words and sentences frequently found together in the training corpus. However, being created by humans, the training data sets can contain significant amounts of social bias and stereotypes, encompassing factors such as gender, race, and religion (Kiritchenko & Mohammad 2018, Nadeem et al. 2021, Stanczak & Augenstein 2021).
Some studies have demonstrated that pretrained language models are capable of acquiring various forms of stereotypical and baised reasoning. For example, Kiritchenko & Mohammad (2018) examined how language models perform in sentiment analysis across various social groups, measuring differences in their behaviours. Recent studies by Nangia et al. (2020) and Nadeem et al. (2021) investigated stereotypical reasoning related to race, gender, profession, and religion using masked language models and sentence encoders.
Recent research examined strategies to reduce the social biases inherent in language models, aiming to enhance their reliability. These studies have investigated techniques to mitigate biases during the learning and prediction phases of language models. Typical methods for mitigating bias involve the use of counterfactual data augmentation (Zmigrod et al. 2019, Dinan et al. 2020, Webster et al. 2020, Barikeri et al. 2021), dropout regularization (Webster et al. 2020), and self-debias (Schick et al. 2021). MIT researchers have trained language models that can realize logic to avoid harmful stereotypes such as gender and racial biases. Luo & Glass (2023) trained a language model to predict the connection between the sentences based on context and semantic meaning. They used data sets with lables for extracted texts showing whether a subsequent phrase “entails”, “contradicts”, or neutral. These data sets were referred as natural language inerence and they found that the logic-based model is considerably lower biased than the previous models.
Furthermore, according to science and technology scholar Winner (2017), language technologies can be regarded as inherently political due to their capacity to drive significant social changes (Winner 2017). Recognizing that language technologies are not only sociotechnical but also fundamentally political, it becomes essential to scrutinize how they prioritize certain perspectives and how their specific design is influenced by the interests and ideologies of particular groups. From an ethical standpoint, the implementation of language technologies requires thoughtful consideration of their inherent biases to prevent any discriminatory effects on marginalized communities.
One of the biases that are causing disadvantages to non-native English speakers can be a detection bias. A research team at Stanford University recently evaluated seven popular GPT detectors by analyzing 91 English essays written by individuals whose native language is not English (Liang et al. 2023). The essays were written as part of the TOEFL exam, and more than 50 percent of the essays were flagged as the production of an AI chatbot. Among the detectors, one program flagged 98% of them as having been composed by AI. On the other hand, when essays written by eighth-grade students whose native language is English and living in the United States were analyzed with the same AI detectors, 90% of the essays were classified as human-generated.
It is surprising that more than half of human generated essasys are categorized as the product of AI. In order to identify the surprising results, the researchers examined the source of discrimination in how the AI detectors distinguish between human and AI-generated contents. According to Liang et al. (2023), AI detectors evaluate “text perplexity”, which measures how “surprised” or “confused” a generative language model is when predicting the subsequent word in a sentence. The text perplexity is considered low if the model can predict the subsequent word easily. On the other hand, vice versa, if the model finds the next word is difficult to predict, the text perplexity is ranked high.
In other words, LLMs like ChatGPT are trained to produce text with low perplexity. However, it can mean that if human writers show limited word choices and use a lot of common words, the detector system can misjudge the text as AI-generated. The risk is much greater with non-native English speakers because they are more prone to use simple words than native English speakers.
Once the researchers identified the bias in the detector programs, they requested ChatGPT to revise the essays using more complex languages and run the edited essays with the detectors again. Surprisingly, these modified essays were all identified as human-authored. Considering these results, as the researchers also noted, one can concern that the GPT detectors overall may encourage non-native English speakers to rely more on GPT or other generative Chatbots in their writings to avoid detection.
Liang et al. (2023) highlighted the seriousness of implications of GPT for non-native English writers and it is imperative to figure out the problem to avoid discrimination. This is especially so because AI detectors could categorise college or job applications as GPT-generated and accuse them of cheating, and this could potentially marginalize non-native English speakers. This could bring about serious consequences on students’ mental well-being.
4. Concluding Remarks
Since the introduction of products like DeepL and ChatGPT, AI-powered language technologies have been steadily advancing towards mainstream acceptance, becoming an indispensable aspect of daily communication and professional routines. Consequently, they play a pivotal role in shaping social interactions and influencing the generation and dissemination of knowledge.
Nevertheless, this dominance presents its own array of challenges, especially to non-native speakers of English. It not only provides limited information to non-native English speakers but also poses the risk of developing a monocultural AI that engages in English but lacking comparably low proficiency in other underrepresented languages. This phenomenon could not only constrain global application of AI, but also create potential cultural biases. Recently, in his blog Gates Notes (2023), Bill Gates discusses how AI is poised to revolutionize computer usage.
He highlights that current software often requires users to navigate through different applications for various tasks, and even the most advanced programs lack a comprehensive understanding of users’ lives. Gates envisions a future where AI agents will enable users to communicate with their devices using everyday language, thus eliminating the need for multiple apps. According to Gates, these programs called AI “agents” will possess a deep understanding of users’ private lives, thus allowing for personalized assistance and streamlining interactions with computers. Gates emphasizes that this development will not only transform user-computer interactions but also revolutionize the software industry (Gates 2023).
What is concerning is these revolutionary benefits of advancement could be only limited to the English native speakers unless appropriate measuers are taken in a timely manner. In order to prevent non-native speakers from being left behind by the native English speakers, it is most important to understand the inequalities to non-native speakers as shown in this study. One potential solution can be sourcing language training data from a wider range of languages, if possible. Or, to make AI tools more relevant and usable in various contexts, localization strategies could also be helpful. In addition, scientists can collaborate with linguists to generate language models that are more linguistically and culturally sensitive. Lastly, another rather radical approach could be intoducing an artificial language into AI chatbot systems to cater to non-native English speakers, which could potentially help reduce inequality. This approach might enhance accessibility and comprehension for individuals who are not fluent in English, thereby promoting inclusivity and leveling the playing field in interactions with AI systems (Park & Tak 2017; Park & Chin 2020; Park 2021, 2022, 2023; Chin 2023). However, it’s crucial to ensure that the artificial language is effectively designed and implemented to accurately convey information and maintain clarity in communication.